- Comments Off on Beyond Off-the-Shelf: How Custom AI Ensures Security and Competitive Edge
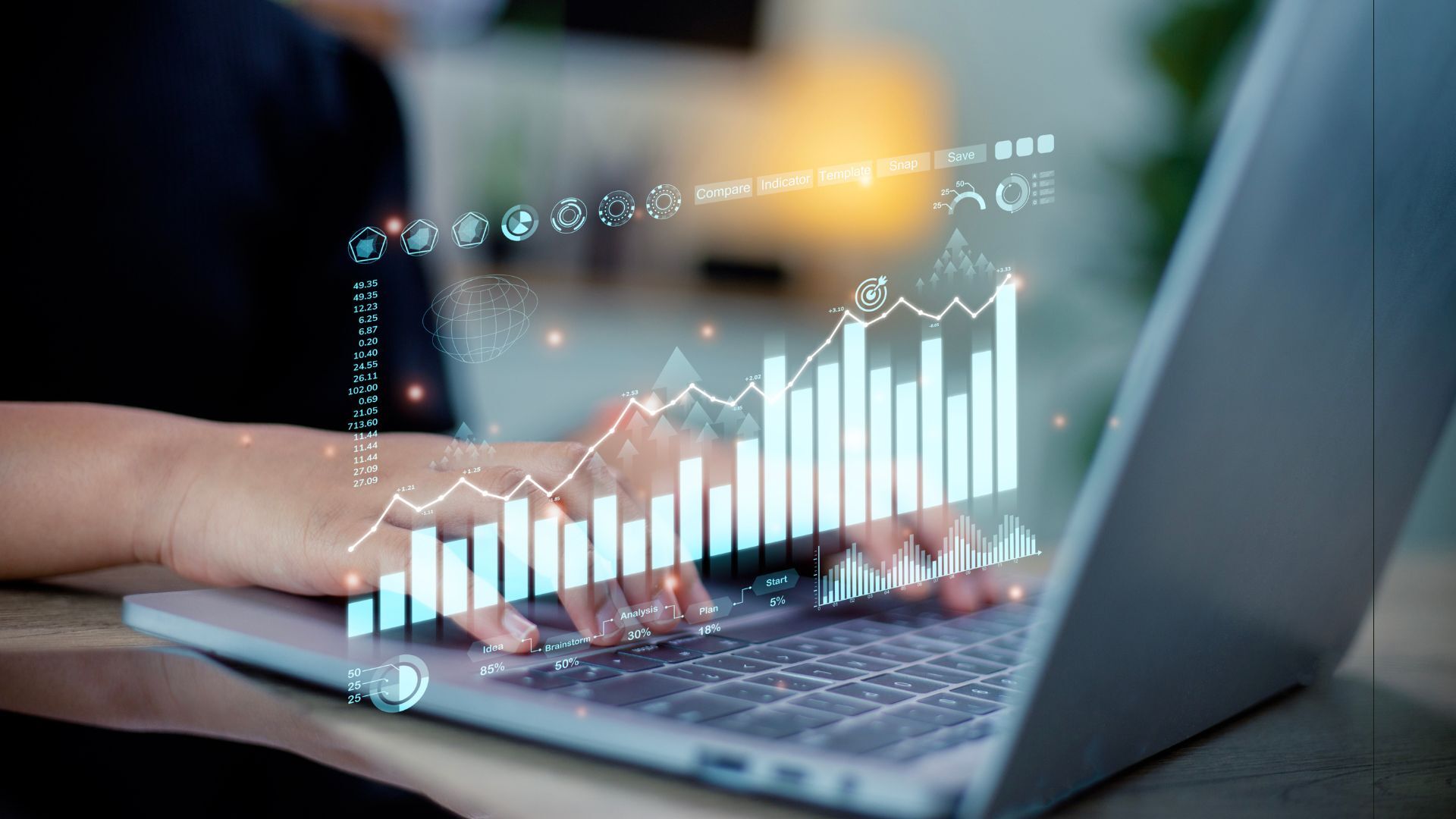
By Giselle Bagatini, Chief AI Officer, Copoly
In the race to adopt AI technologies, many businesses eagerly embrace public large language models (LLMs) like ChatGPT. While these tools offer impressive capabilities, they also introduce significant risks that companies often overlook. As a data scientist and AI consultant, I’ve witnessed how indiscriminate use of public AI models can jeopardize data security, intellectual property (IP), and regulatory compliance. Additionally, public models often struggle with hallucinations—generating factually incorrect or misleading information. It’s time for businesses to consider the benefits of custom LLMs to mitigate these challenges.
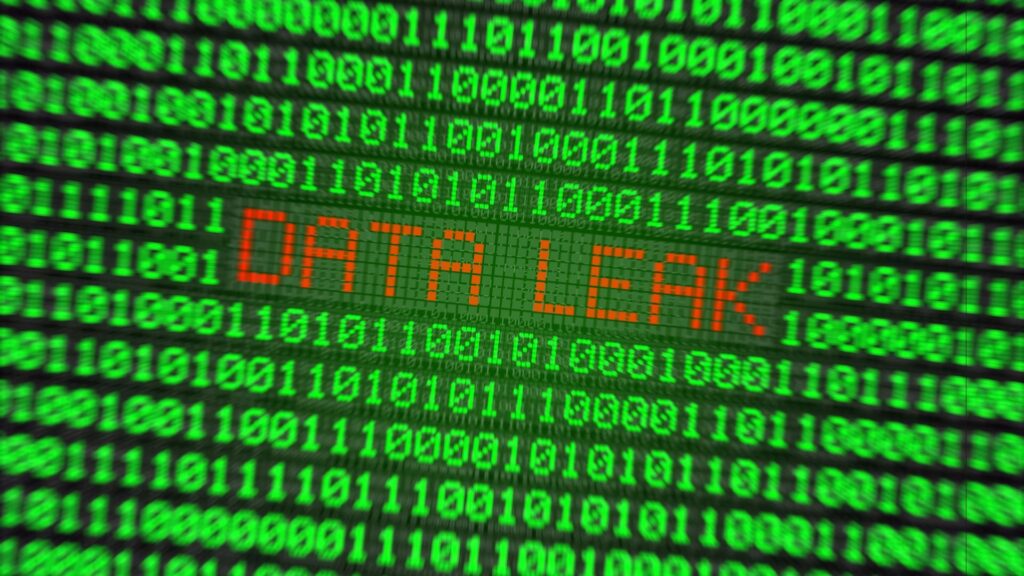
The Pitfalls of Public LLMs
The appeal of off-the-shelf AI models is undeniable: they’re accessible, require minimal setup, and can handle a wide range of tasks from content creation to data analysis. However, this convenience comes at a cost:
- Data Security Risks: Every interaction with a public AI model sends data to third-party servers, increasing the risk of data breaches and unauthorized access to sensitive information.
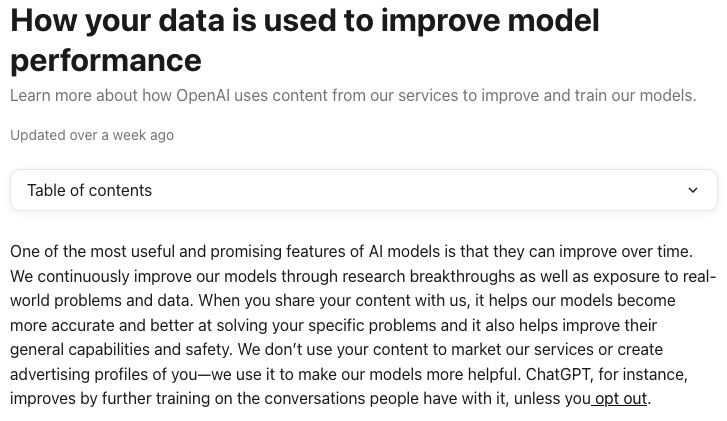
- Intellectual Property Vulnerabilities: When proprietary information is entered into a public model, trade secrets and competitive advantages may be exposed.

- Compliance Nightmares: In industries like healthcare and finance, strict regulatory requirements (e.g., HIPAA, GDPR) may be inadvertently violated when sensitive data is processed through public AI platforms.
- Hallucinations and Inaccuracies: Public models can generate incorrect or misleading information. This risk is especially problematic in industries that require high precision, such as legal services or healthcare. Retrieval-Augmented Generation (RAG) helps mitigate this by grounding AI outputs in real, trusted data sources.
- Lack of Specialization: General-purpose models often struggle with industry-specific terminology and context, leading to less relevant outputs.
The Custom LLM Advantage
Custom large language models, tailored to your business, offer a compelling alternative to public AI models:
- Enhanced Security and Control
By developing and hosting your own LLM, you retain full control over your data within your organization’s infrastructure, drastically reducing the risk of data leaks and safeguarding your intellectual property.
- Improved Accuracy and Relevance
Custom LLMs trained on your industry-specific data understand your unique processes and terminology, ensuring more accurate outputs and better decision-making support.
- Compliance Assurance
Custom models give you full control over the data used for training and inference, making it easier to comply with regulations like GDPR, HIPAA, and other industry-specific standards.
- Long-Term Cost Efficiency
Although custom LLMs require higher initial investments, they can be more cost-effective over time by eliminating subscription fees for public models and providing enhanced accuracy tailored to your business needs.
Implementing a Custom LLM
Successfully implementing a custom LLM requires careful planning:
- Define Clear Objectives: Identify specific tasks where a custom AI can add the most value.
- Gather Quality Data: Collect and curate relevant, high-quality data for training.
- Choose the Right Technology: Select appropriate base models and fine-tuning techniques.
- Prioritize Security: Implement robust encryption and access controls to protect your data and model.
- Ensure Ethical Use: Develop guidelines to prevent bias and ensure fair, responsible AI use.
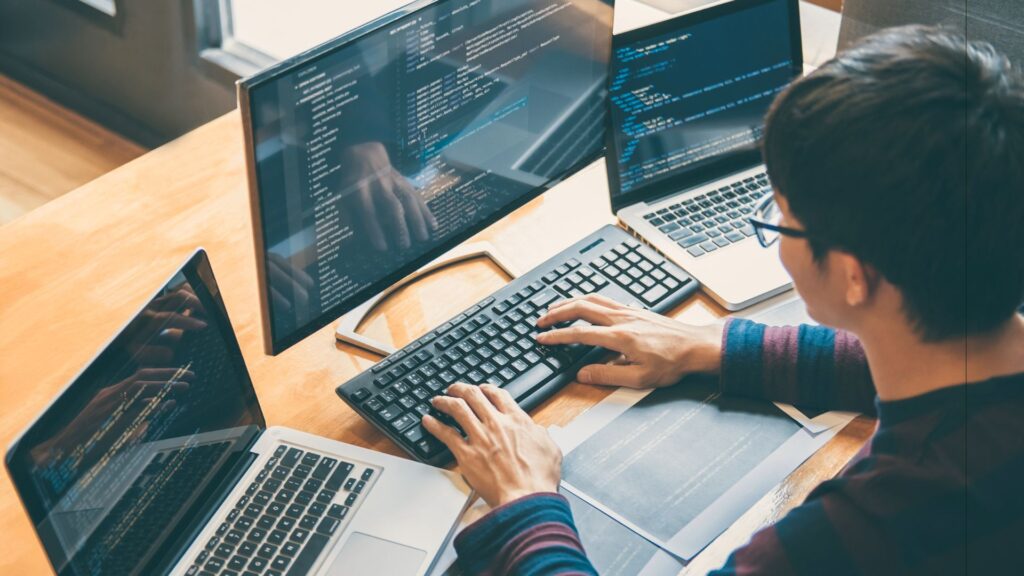
The Competitive Edge of AI Customization
According to a McKinsey & Company report, top-performing companies in AI adoption are more likely to customize their AI models rather than relying solely on off-the-shelf solutions. These leaders integrate AI across multiple business functions while focusing heavily on risk mitigation, particularly in cybersecurity and IP protection.
“High performers are much more likely than others to follow a set of risk-related best practices,” notes McKinsey, emphasizing the importance of secure and compliant AI adoption strategies(McKinsey).
Real-World Example: AI in Cancer Support
Recently, we worked with a healthcare client to develop a custom AI chatbot aimed at supporting cancer patients. To ensure personalized, accurate responses, we incorporated a powerful technique called Retrieval-Augmented Generation (RAG) alongside a generative AI model.
- What is Retrieval-Augmented Generation (RAG)?
RAG combines generative AI with a retrieval system. Instead of relying solely on pre-trained knowledge, the AI pulls real-time information from a curated database (such as PDFs, documents, or trusted websites). This approach ensures that the chatbot’s responses are both contextually relevant and factually accurate, grounded in verified, up-to-date information.
For example, when a patient asks a question, the generative AI formulates an empathetic, personalized response, but the RAG framework ensures the AI draws from trusted medical sources. This dual approach enhances both the quality and reliability of the interactions, providing much-needed reassurance in sensitive health matters.
- Enhanced Data Security and IP Protection
In addition to improved accuracy, using a custom LLM with RAG ensures data privacy. Unlike public AI models that require sending information to external servers, this solution operates in-house, allowing the client to control their proprietary data. Sensitive patient interactions and medical records are securely handled within the organization, reducing the risk of data leaks.
- Why Customization Matters
Generic AI models, while powerful, lack the depth needed for specialized fields like healthcare. By fine-tuning the model with industry-specific data and incorporating RAG, businesses gain a tailored solution capable of delivering high-quality, domain-specific interactions—far superior to what a generic, off-the-shelf model can provide.
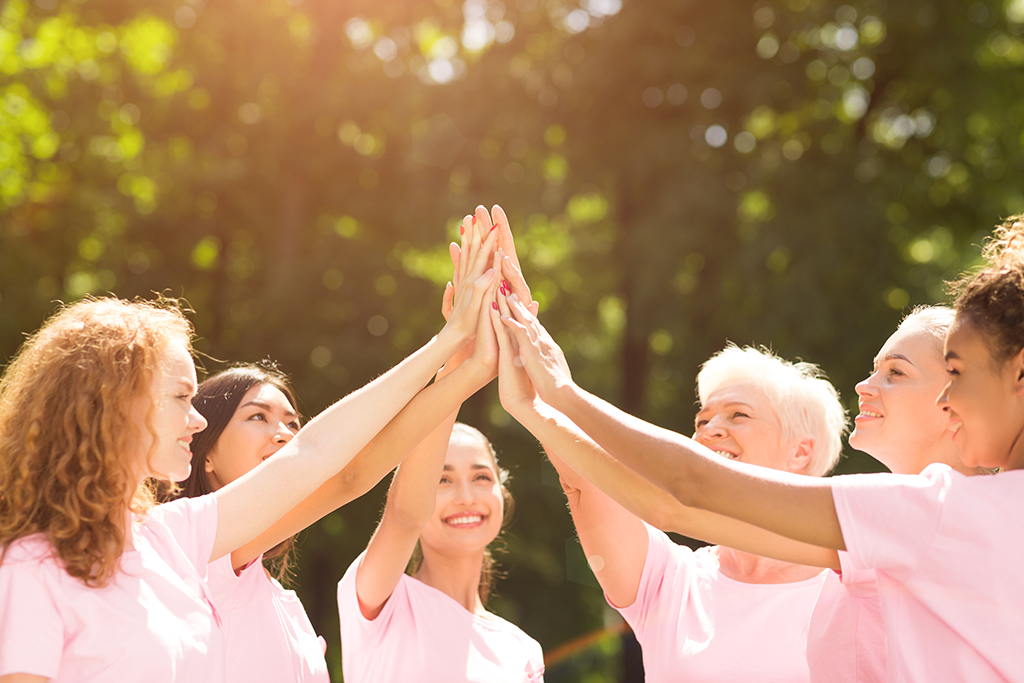
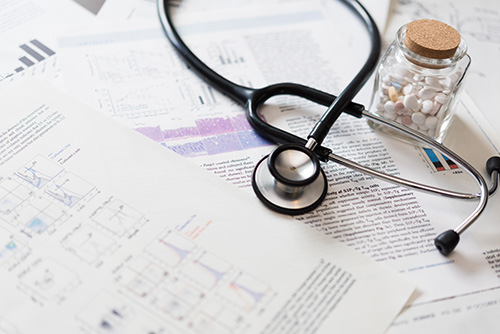
Real-World Example: BioSage by Copoly
We recently developed BioSage, a custom AI tool for summarizing scientific literature in bioinformatics. To ensure precision, BioSage integrates (RAG) with accustom fine-tuned generative AI model and framework, pulling real-time data from trusted sources like academic papers and curated databases. This ensures that BioSage’s summaries are not only informative but grounded in accurate, up-to-date research.
For example, when a researcher queries BioSage, the generative AI provides a concise, context-aware summary, while RAG verifies the content against scientific sources. This reduces the risk of hallucinations, and guarantees factual accuracy, essential for scientific analysis.
Using BioSage, Copoly’s custom solution ensures data privacy, handling proprietary research data securely within the organization. Fine-tuned for bioinformatics, BioSage offers high-quality, domain-specific insights, far exceeding the capabilities of off-the-shelf models.
Conclusion: A Strategic Imperative
As AI continues to reshape the business landscape, choosing between public and custom LLMs has become a critical strategic decision. While public models provide a quick entry point, they expose businesses to significant risks and limitations.
Custom large language models represent a more mature, secure, and effective approach to AI adoption. They offer enhanced security, precision, and long-term value that drive innovation and maintain a competitive edge.
In today’s data-driven economy, protecting sensitive information while harnessing its full potential is crucial. Custom LLMs allow businesses to do just that—turning AI from a potential liability into a powerful, secure asset.
The future belongs to those who can harness AI’s power responsibly and effectively. For forward-thinking businesses, the path to that future is clear: it’s time to go custom.
Ready to take your AI strategy to the next level? At Copoly, we specialize in creating custom AI solutions that enhance security, accuracy, and efficiency for your business. Whether you’re looking to fine-tune a tailored large language model or need help navigating complex AI challenges, our team is here to guide you. Contact us today to learn how we can develop secure, cutting-edge AI models that meet your industry-specific needs and give you a competitive edge.
Related Post
Unlocking the Future of Cancer Therapy: CRISPR/Cas Meets Copoly
- Comments Off on Unlocking the Future of Cancer Therapy: CRISPR/Cas Meets Copoly
EVO-2: Revolutionizing Biomedical Research with AI-Driven Genomics
- Comments Off on EVO-2: Revolutionizing Biomedical Research with AI-Driven Genomics
Preparing for AI in Genetic Engineering R&D: A Roadmap for Success
- Comments Off on Preparing for AI in Genetic Engineering R&D: A Roadmap for Success